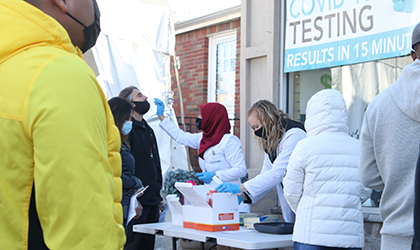
What to do about Covid? With nearly 60 million cases and 1.4 million deaths worldwide as of the end of November 2020, there are still no consistently effective treatments or approved vaccines yet (though large-scale vaccine trials have already produced promising results). Social distancing, mask wearing, and infection control practices can reduce the rate of spread somewhat, but as long as infectious individuals circulate amongst susceptible persons, continued spread is inevitable, given that most populations have not built immunity against SARS-CoV-2 to any meaningful extent.
Halting transmission requires identifying infectious persons and isolating them from those who remain susceptible to the virus. Repeatedly testing individuals for SARS-CoV-2 is the key step towards achieving this end.
While public health professionals recognize the importance of aggressive screening and isolating those testing positive to control coronavirus outbreaks, many citizens and politicians do not (though President-Elect Joe Biden lists testing as his first priority in gaining control of Covid-19). One normally seeks diagnostic testing for personal health reasons: on the basis of a test, you change your diet, or are prescribed medication, or are even directed to have surgery. Covid testing is different – the reason to be tested is to protect those around you from you if you are infected, rather than for your own immediate benefit.
A simple model makes this clear. The black curve in Figure 1 displays the rising and then falling rate with which an infected person or index transmits new infections to others dependent upon the duration of time since the infection of the index; such curves have been estimated statistically based on observed outbreaks such as in Wuhan.1 The average number of infections transmitted by the index is the well-known reproduction number R0 (“R naught”) that has emerged from its home in epidemiology textbooks into our daily conversation and nightly news broadcasts; it is equal to the area under the entire curve in Figure 1.2
Now imagine that thanks to the result of an accurate Covid test, the index is isolated at some time, say T days after infection. The isolation brings transmission to an early end, reducing the average number of infections from the area under the entire curve in Figure 1 to just the red area in the figure. Isolating the index has blocked all transmission associated with the gray area of the curve. Aggressive screening makes it possible to amplify these effects by repeatedly identifying and isolating infectious individuals.3
Figure 1. The black transmission curve shows the rate of transmission of new infections by an index infected at time 0. If the index receives a positive test and is isolated at time T, further transmissions are blocked so that the total expected number of transmissions is reduced from the full area under the transmission curve to the red area.
Testing and isolation can be made most effective by minimizing the time from infection until those infected are identified and isolated. Several factors affect the time until transmission can be interrupted, some controllable but others less so, as discussed below.
First, it is important to use a test that detects active versus past infection. This is why diagnostic polymerase chain reaction (PCR) tests that detect coronavirus ribonucleic acid (RNA) indicative of active infection are the most useful tests for interrupting transmission. Antibody tests that report whether a person was previously infected are not appropriate for this purpose. Even PCR testing cannot always detect infected individuals. The sensitivity of a test is the probability that an infected person would register a positive result upon testing. As shown in Figure 2, recent statistical analysis estimates that the sensitivity of PCR tests is close to zero for the first few days after infection, increases with the passage of time since the virus is acquired to a maximum of just over 80% around 8 days after infection, and then declines as the time from infection increases further.4 This result suggests that at least three days from infection must pass before a PCR test is likely to produce a positive result.
Figure 2. The evolution of test sensitivity over time since infection as estimated by Kucirka et al.4 The vertical scale reports test sensitivity, which is the probability an infected person will test positive as a function of the number of days that has passed since the time of infection.
Interrupting the transmission curve also depends upon the timing of the testing process. Most Covid tests worldwide have been administered to persons who experience symptoms, such as fever, a sore throat, or losing the sense of taste or smell. Those tested symptomatically must first experience symptoms, which makes test timing dependent upon the incubation time from infection to symptoms, estimated statistically as about 5.2 days on average with a standard deviation of just under 4 days.1 This would be the minimum time after infection at which a test might be administered, for even if one self-recognizes Covid symptoms, another few days could pass before testing is sought, and as discussed earlier, the PCR test must detect the infection. The incubation time also provides a minimum time estimate for when symptomatic individuals could be expected to take steps towards preventing transmission to others, as public health authorities recommend that such persons stay at home except to receive medical care, whether or not they have been diagnosed with Covid-19. Some individuals might resist self-isolation without receiving a confirming positive test result. For such persons, isolation is further delayed by the time required to receive the positive test result.
Another path to testing and isolation is based on contact tracing, whereby an index who has tested positive is interviewed to determine others with whom they interacted during the previous week or so.1 A contact who was referred (and actually infected) by the index cannot be tested until they have been notified (a process that can easily take a week or more) and testing can be arranged. Even without testing, a contact could quarantine and, if infected, further transmission could be blocked, but only after the contact has been notified.
Asymptomatic testing of the population without regard to symptoms is a direct attempt to identify infectious individuals and isolate them to prevent further spread. This approach is particularly well-suited for population groups consisting of persons thought to be at high-risk of infection. A notable recent setting for asymptomatic testing is repeat screening of residential college students. A small number of US universities including Harvard, MIT, Yale, and the University of Illinois have embarked upon twice weekly screening of college students to try and circumvent outbreaks on campus. In addition to the evolution of test sensitivity (Figure 2) and the delay from sample collection until results are obtained and students are instructed to isolate, the time at which transmission can be interrupted in such repeat testing programs critically depends upon the frequency of testing. Twice weekly testing will identify infected students more quickly than weekly testing, which in turn will find infections faster than biweekly screening. Combining all of these factors to determine the probability of interrupting transmission at various times after infection is key to evaluating the effectiveness of any repeat testing proposal.
Repeat testing on campus
We developed a model to help Yale University plan its repeat testing program this semester.3 The model accommodates different statistically estimated curves for the rate of coronavirus transmission with time from infection. In particular, in addition to the curve shown in Figure 1, we also consider transmission curves that feature earlier versus later transmission. Early transmission is more difficult to control; given the inevitable lag from infection until detection and isolation, more transmission will already have happened by the time of isolation with early transmission curves. The model allows different choices for the evolution of test sensitivity (of which Figure 2 is an example), screening frequencies, and delays from testing until isolation for those who test positive. The model also lets users specify different student population sizes, initial numbers of infected students at the start of the school year, the importation of infections from off of the college campus into the student population, different planning horizons, and the reproductive number characterizing student behavior in the campus context. A publicly available web application enables users to design their own testing programs under alternative assumptions to see how many infections are estimated to ensue. The program also calculates the number of students in isolation over the planning horizon, including those students isolated on account of false positive tests. University public health experts agreed that keeping the total number of student infections below 5% of their population constituted a reasonable public health goal.3 Using the model, we were able to determine the worst transmission scenarios that satisfied this goal for different testing frequencies. This analysis suggested that twice weekly testing was necessary for undergraduate students given their potentially high transmission potential as expressed via reproductive numbers; graduate and professional students were judged to behave in less risky fashion, enabling a weekly testing benchmark for that group.
American universities welcomed students back to campus this past summer, but to mixed results. Whether due to cost or complexity, very few universities have instituted intensive repeat testing programs, and as feared by many, several colleges have experienced major outbreaks. The New York Times has been tracking college Covid-19 case statistics since the summer, and reports more than 321,000 cases among college students as of the end of November 2020, while a report from the US Centers for Disease Control noting the recent increase in Covid-19 cases among persons aged 18-22 years states that: “Because approximately 45% of persons aged 18–22 years attend colleges and universities … it is likely that some of this increase is linked to resumption of in-person attendance at some colleges and universities.” In the United Kingdom, more than 35,000 university students have tested positive for Covid-19 as of the end of November 2020, though this is also thought to be an underestimate.
Figure 3 reports the daily percentage of the student body testing positive for three well-known American universities: Notre Dame, the University of Illinois at Urbana-Champaign, and Yale. Notre Dame initially screened students as they arrived on campus but did not implement repeat testing afterwards. They experienced a very large outbreak in mid-August with 273 of 12,780 students testing positive from 17-19 August. This led that university to retreat to online-only instruction until 2 September. The University of Illinois sponsored a repeat saliva testing program with all students tested twice weekly. As widely reported however, students who knowingly tested positive still attended parties and other extracurricular university events, with 653 of 49,000 students testing positive over the last four days of August alone. Several students were disciplined for breaking university rules and a campus-wide quarantine was imposed, enabling the university to regain control of its outbreak. This example shows that simply testing frequently has no effect unless those found infected are isolated from the rest of the university population.
Transmission during superspreading events is also difficult to prevent via repeat testing, though frequent testing serves to halt further infection after the event. By way of illustration, as of 11 October 2020 at Yale, only 24 positive tests were scattered randomly over time among the 5,000 students being screened regularly. This changed dramatically when 6 and then 10 students tested positive on 12 and 13 October. These students were all members of the Yale men’s hockey team, all of whom had tested negative as recently as Friday, 9 October, the date of their last practice. As announced in a 15 October alert to the university, an additional two players tested positive, bringing the total number infected up to 18 out of 27 team members. In addition to immediately isolating and further testing the entire team and staff, contact tracing resulted in the quarantine and testing of reported non-team close contacts, all students were advised to self-identify whether they had been in close contact with any members of the hockey team over the prior 7 days and if so to quarantine until regular testing yielded a negative result, all sports teams were instructed to cease in-person training, several athletic facilities were closed for cleaning, and the Covid-19 alert level was raised from green to yellow, signaling an increase in transmission risk on campus. Quite by coincidence, a Centers for Disease Control report issued on 16 October reported another Covid-19 outbreak associated with a hockey game. That report states that: “The ice rink provides a venue that is likely well suited to Covid-19 transmission as an indoor environment where deep breathing occurs, and persons are in close proximity to one another.” Even frequent asymptomatic testing cannot stop superspreading transmission from occurring. Indeed, to block such transmission would require preventing superspreading events in the first place, some of which may be difficult to identify before the fact. Nonetheless, Yale just completed the in-residence part of the fall 2020 semester with a total of 154 positive tests among the 5,000 undergraduate and graduate students in residence, well within the previously agreed-upon safety level of 5% total student infections.
Figure 3. Percentages of students testing positive at three universities in Fall, 2020. (Note: an earlier version of this figure incorrectly referred to “proportions” instead of “percentages” of students. This has now been corrected.)
Repeat testing off campus
Outside the university setting, repeat asymptomatic testing in the general population has not taken hold. Part of the reason is that public testing capacity is severely constrained, and federal and state government officials have continued to emphasize symptomatic testing. One proposal for implementing asymptomatic testing has been to focus on high priority populations such as workers and residents in nursing homes, or those living in high density congregate housing, identify a discrete number of testing sites, and then test aggressively in those locations felt to be at highest risk.5 However, the proposal comes with a statistical call for random sampling to ascertain the population-wide level of infection. While of interest in its own right, in the context of aggressive community screening, the overall population level of infection can also be used to guide more targeted testing. Given a testing focus in those locations whose populations are at highest risk of infection, if the fraction of infected persons identified in any of these sites falls substantially below the population average as deduced from random sampling, then underperforming sites relative to the random sample should be abandoned in favor of as yet untested locations believed to be high risk a priori. In this way, the statistical random sample can be used to drive targeted asymptomatic testing towards those locations with higher-than-average test positivity results, preventing more infections in the process.
However, testing alone will not prevent infections if those found infected cannot be isolated. In certain populations, especially persons of very limited means, it is likely that home isolation will not prove practical – one cannot expect an individual testing positive to isolate when three families are sharing two rooms in one apartment, for example. Preventing infections via testing and isolation in such a circumstance obligates the government to provide public assistance in the form of isolation quarters, and perhaps even payments akin to what workers receive while serving mandatory jury duty, to help enable at least some isolations to take place in the service of preventing further transmission and preserving the public’s health.
Testing to isolate infectious individuals represents our best hope at stemming the spread of coronavirus while the world waits for effective vaccines. Statisticians have important roles to play in helping to explain how such programs can prevent infections and which testing approaches make the most sense in different infection risk environments.
Acknowledgment
The authors thank Tim Pavlis, associate vice president for strategy and academic business operations at Yale University, for curating the data underlying the analysis of Figure 3.
About the authors
Joseph T. Chang is professor of statistics and data science in the Department of Statistics and Data Science, Yale University.
Edward H. Kaplan is William N. and Marie A. Beach professor of operations research, professor of public health, and professor of engineering in the Yale School of Management.
References:
- Kaplan, E. H. (2020) Containing 2019-nCoV (Wuhan) coronavirus. Health Care Management Science, 23, 311–314. https://doi.org/10.1007/s10729-020-09504-6
- Champredon, D. and Dushoff, J. (2015) Intrinsic and realized generation intervals in infectious-disease transmission. Proc. R. Soc. 282:20152026. https://doi.org/10.1098/rspb.2015.2026
- Chang, J. T., Crawford, F. W. and Kaplan, E. H. (2020) Repeat SARS-CoV-2 testing models for residential college populations. Health Care Management Science. https://doi.org/10.1007/s10729-020-09526-0
- Kucirka, L. M., Lauer, S. A., Laeyendecker, O., Boon, D. and Lessler, J. (2020) Variation in false-negative rate of reverse transcriptase polymerase chain reaction-based SARS-CoV-2 tests by time since exposure. Annals of Internal Medicine, 173(4), 262-267. https://www.acpjournals.org/doi/10.7326/M20-1495
- Kaplan, E. H. and Forman, H. (2020) Logistics of aggressive community screening for coronavirus 2019. JAMA Health Forum. https://jamanetwork.com/channels/health-forum/fullarticle/2765693