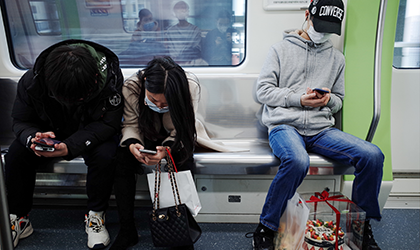
In order to answer the most pressing questions we have about Covid-19 – how quickly will it spread, how many hospitalizations will be required, how many people may die – we first need to know how many people are infected today. Knowledge of the current prevalence of infection can be incorporated into a model such as the SIR model to project health care needs, as the University of Pennsylvania’s CHIME app does. Unfortunately, the data we have only tells us the number of confirmed cases, not the total number of infections.
There are multiple reasons that we haven’t observed every infection. Some people who become infected develop mild or even non-existent symptoms (but are still able to pass the virus on to others), while others who do develop serious symptoms are not able to get tested due to test shortages. Raw tallies of diagnosed infections, then, are not precise measurements of the number of infected people, but instead reflect some combination of the number of infections in the population, the rate of testing in the population, and the proportion of infections that cause symptoms. In order to estimate the total number of infections, scientists must fit what data they do have into a model that can account for the incompleteness and bias introduced by the testing environment.
Several recent papers attempt to do exactly that. By reviewing their methods and assumptions, we can gain some insight into how scientists make defensible inferences given the incomplete and possibly biased data they have available.
Perkins et al. aim to estimate the total number of infections in the United States, as of 12 March. They do this by modeling the spread of the disease in two parts. “Imported infections” refer to people who arrive to the United States with the disease, while “locally transmitted infections” refer to those who acquire the disease domestically.
To estimate the number of imported infections, they use data on deaths from imported infections. How do they get from deaths to total infections? They look at existing research about the proportion of symptomatic infections that result in death, called the “case fatality rate”, to go from number of recorded deaths to number of symptomatic infections. This allows them to estimate that about 39% of imported symptomatic infections are actually reported. They then look to existing research on the proportion of infections that are symptomatic to get from number of symptomatic infections to total number of infections. By plugging in a range of evidence-informed inputs, they get a range of possible values for the total number of imported infections for each day.
In order to estimate locally transmitted infections, Perkins et al. incorporate their estimates of imported infections into a SIR model, similar to the one that Ball describes in his article, that models the spread of the disease day by day. They rely on existing research and observations in other countries to inform the other inputs to the model. In all, they estimate that between 7,451 and 53,044 people in the US had been infected as of 12 March.
Similar to Perkins, Johndrow et al. also use observed deaths and existing research about the fatality rate of Covid-19 to work back to the total number of infections. Relying on clinical results to model the time lag between infection, symptom onset, and death, they assemble a SIR model to simulate the spread of the disease and fit it to the daily death totals. This allows them to infer the remaining parameters, including the size of the infected population. Why take this particular approach? As the authors state, numbers of deaths caused by Covid-19 have “a reasonable chance of being measured correctly”. Given all of our caveats about using the unadjusted number of positive tests when making inferences, the completeness of available data is clearly an important consideration when selecting an appropriate model. They estimate that around 475,000 people in the United States had been infected by 18 March.
Unlike Perkins and Johndrow, Verity et al.’s primary question is not to estimate the number of undetected infections, but rather two key measurements of the deadliness of Covid-19: “case fatality rate”, the proportion of symptomatic infections that result in death, and “infection fatality rate”, the proportion of all infections resulting in death. Though they have a different primary question, Verity et al. recognize that “surveillance of a newly emerged pathogen is typically biased towards detecting clinically severe cases, particularly at the start of an epidemic” and estimates of fatality “may thus be biased upwards until the extent of clinically milder disease is determined”. So they too must find a way to estimate the number of undetected infections in order to proceed.
Verity et al. note that recorded cases from the Chinese city of Wuhan – site of the original reported coronavirus outbreak – are much more severe than those from other parts of China. They also note that older people are over-represented in recorded Covid-19 cases relative to their proportion of the population – this is somewhat true in the rest of China and especially so in Wuhan. The authors reason that both of these facts reflect the same dynamic – the observed data are biased towards more severe cases because that’s who is getting tested. Since older people are more likely to experience severe symptoms, we should expect the data to be biased towards older people. In Wuhan, testing was only happening in hospitals, so the bias is most severe. In the rest of China, testing was expanded to travelers as well as others through contact-tracing, and so there was increased representation of milder cases.
Verity et al. then make a couple of key assumptions. They assume that rates of symptomatic infection are roughly the same across age groups, so that observed differences in rates of positive tests are entirely due to the bias described above. They further assume that all symptomatic cases in the 50-59 age range for people in China outside of Wuhan were observed (100% reporting). With that, they are able to estimate the amount of relative under-reporting by age range, in Wuhan and in the rest of China. These estimates of reporting bias allow them to adjust the number of observed symptomatic cases to account for the under-reporting, and get to a more accurate denominator when calculating the case fatality rate. They arrive at an overall range of 1.2%-1.5%, but with substantial variation among age groups and much higher rates for older people.
To account for asymptomatic cases, the authors use data on international residents of Wuhan who were repatriated during the outbreak. In this population every individual was tested, making possible an estimate of what proportion of infections have no symptoms. With this additional piece, they can calculate the infection fatality rate. They estimate a 95% credible interval of between 0.4% and 1.3% overall, once again with much higher rates for older populations.
Both of these estimates rely on the strong key assumptions described above. Data from the Diamond Princess cruise liner provides evidence to support the modeling choices the authors made. The Diamond Princess population, like the Wuhan repatriation population, is one in which every individual was tested. Verity et al. observe that the measured infection fatality rate on the Diamond Princess is in line with estimates from their model.
We’ve seen three different studies that touch on the question of how many people are infected. Perkins and Johndrow both rely on existing research about the fatality rate of Covid-19 and the observed number of deaths to get to an estimate of the total number of infections. Verity et al., for whom the fatality rate is itself the quantity they want to estimate, rely on some key assumptions about susceptibility for different age groups in order to estimate rates of under-reporting, and thus arrive at the total size of the infected population. These papers also reveal how researchers evaluate the quality of data as it relates to their research question. The authors of Johndrow rely heavily on observed deaths by Covid-19 because they believe that data is more complete than numbers of positive Covid-19 tests. Verity et al. rely on the Wuhan repatriation data, one of the few instances where the entirety of a susceptible population was tested, for information about asymptomatic cases.
As time goes on and scientists continue to study the SARS-CoV-2 virus and its disease, Covid-19, they’ll incorporate new knowledge into their models, making them better. More data, from more varied contexts, will allow them to make better inferences with those models. As that process plays out, the rest of us who want to stay informed should pay attention to the models, assumptions, and data that go into the numbers we see. We should pay attention to what key assumptions researchers are making as well as how well the models explain the data we have observed.
Acknowledgements
I am grateful for comments and feedback provided by Patrick Ball and Megan Price.
About the author
Tarak Shah is a data scientist at the Human Rights Data Analysis Group (HRDAG), where he processes data about violence and fits models in order to better understand evidence of human rights abuses.