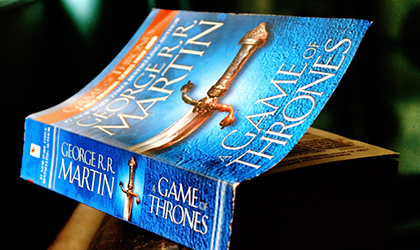
Five volumes were published between 1996 and 2011, and there are two more still to come. Waiting fans have been feverishly speculating about how the cliff-hangers at the end of book five, A Dance with Dragons, will be resolved in the next book, The Winds of Winter. Publication of that novel is still not set in stone, but those wondering about the fate of (no spoilers) the character we’ll refer to as X have only days left to wait for a resolution. Season five of the TV show ended on the same note of uncertainty as book five, but season six begins on Sunday, 24 April.
Rather than waiting patiently, I wondered whether I could make a data-driven prediction about what might happen next based on an analysis of the books. Several of Martin’s characters, including the red priestess Melisandre, the greenseers of Westeros, and the Warlocks of Qarth, seem to have the ability to foretell the future. Lacking access to their techniques, I fell back on Bayesian statistics.
What fate awaits?
Each of the chapters in Martin’s novels is written from the third-person point-of-view perspective of one of his characters, and the number of chapters each character receives varies between books. My idea was to use the distribution of these chapters in past novels to make predictions for the number of chapters each character might receive in the next series instalment.
Happily, dedicated fans at the website lagardedenuit.com (meaning “The Night’s Watch”, one of many factions in the series) had counted up the number of point-of-view chapters per character per book, and had tabulated it in a 24×5 matrix. In statistics, this is known as panel data, and a standard technique for analysing it is to use a hierarchical model. In a hierarchical model, you assume that each character’s point-of-view chapters follow their own trend, but these trends are controlled by some underlying structure.
In the chosen model, the point-of-view chapters for individual characters follow a Poisson distribution (there being too little data for a more complicated model), but characters enter and leave the action at times which are themselves normally distributed. This produces a model with six parameters. An algorithm called Gibbs sampling (which involves stepping through the parameters one at a time and taking a sample while holding all the other parameters fixed) can be used to fit the model and produce predictions.
Figure 1 shows the predictive distribution for the number of point-of-view chapters appearing in the next book for one of the characters, Sansa Stark. The single likeliest value is 0, but it is misleading to take this as our prediction, as it is even more likely that there will be some positive number of chapters. The best way to summarise this distribution is a statement like “either 0 or 3–7 chapters”.
Figure 1. A predictive distribution for the number of point-of-view chapters for the character Sansa Stark in The Winds of Winter.
Of most interest to me, of course, was the prediction for character X, who was left in a very difficult position at the end of book five (and season five). Will this be the end, or can we expect a “with one bound, Jack was free” scenario? The model suggests that X has a 60% chance of having at least one point-of-view chapter in the next book, so my money should be on survival – although my knowledge of the book, together with Martin’s fondness for killing off fan favourites, suggests that X is doomed.
A tricky business
Ice and Fire fans have pointed out various flaws in the model, including that it ignores most details of the story. Some of these details could have been incorporated by using informative priors, but in other cases this seems to be very difficult. Data scientists were less uniform in their opinions, with comments ranging from “simplistic” to “cute” to “over-fitted”. But anyone who has used a naive Bayes classifier knows that the wrong model can still produce good predictions. In this case, however, I agree with the fans, and made a list of some of the problems with the model in my paper, which is available online. Of course, we should attempt to validate the model by seeing what predictions it would have made for earlier books, but this leads to no firm conclusions.
There is also the question of how to measure the predictive performance of the model once the next book is published. Since the model’s predictions are subjective probabilities, it is hard to know how to check that they are right. However, I am confident that we will be able to see whether the model is very wrong, and I hope to investigate this in a future sequel or addition to the paper.
Until the model has been put to a real-life test, the work is only half done. As Martin himself stated (according to westeros.org) in a 2002 interview: “Prophecy can be a tricky business.”
- Richard Vale has a PhD in mathematics from the University of Glasgow and was an HC Wang Assistant Professor at Cornell University. He has recently been a lecturer in statistics at the University of Canterbury, New Zealand, and continues to be involved in applied research. He is currently a senior intelligence analyst in the public sector. For further spoiler-free information, see here.
- This is an updated version of an article originally published in the December 2014 issue of Significance Magazine. Read the full issue, for free, online or via our new iOS and Android app.